报告主题:A Unified Framework for Non-Convex Low-Rank Matrix Recovery Problems
主讲人:蔡剑锋 教授(香港科技大学)
主持人:尹国建 研究员
时 间:2023年10月20日周五16:00
地 点:致知楼706
报告摘要:
The challenge of recovering low-rank matrices from linear samples is a common issue in various fields, including machine learning, imaging, signal processing, and computer vision. Non-convex algorithms have proven to be highly effective and efficient for low-rank matrix recovery, providing theoretical guarantees despite the potential for local minima. This talk presents a unifying framework for non-convex low-rank matrix recovery algorithms using Riemannian gradient descent. We demonstrate that numerous well-known non-convex low-rank matrix recovery algorithms can be considered special instances of Riemannian gradient descent, employing distinct Riemannian metrics and retraction operators. Consequently, we can pinpoint the optimal metrics and develop the most efficient non-convex algorithms. To illustrate this, we introduce a new preconditioned Riemannian gradient descent algorithm, which accelerates matrix completion tasks by more than ten times compared to traditional methods.
嘉宾简介:
蔡剑锋是香港科技大学数学系教授。2000年和2004年分获复旦大学学士和硕士学位,2007年获香港中文大学博士学位。博士毕业之后,他曾先后在新加坡国立大学,美国洛杉矶加州大学,和美国爱荷华大学工作,直到2015年加入香港科技大学。他的研究兴趣是信号、图像和数据的理论和算法基础。他在2017年和2018年被Clarivate Analytics评选为全球高被引学者。
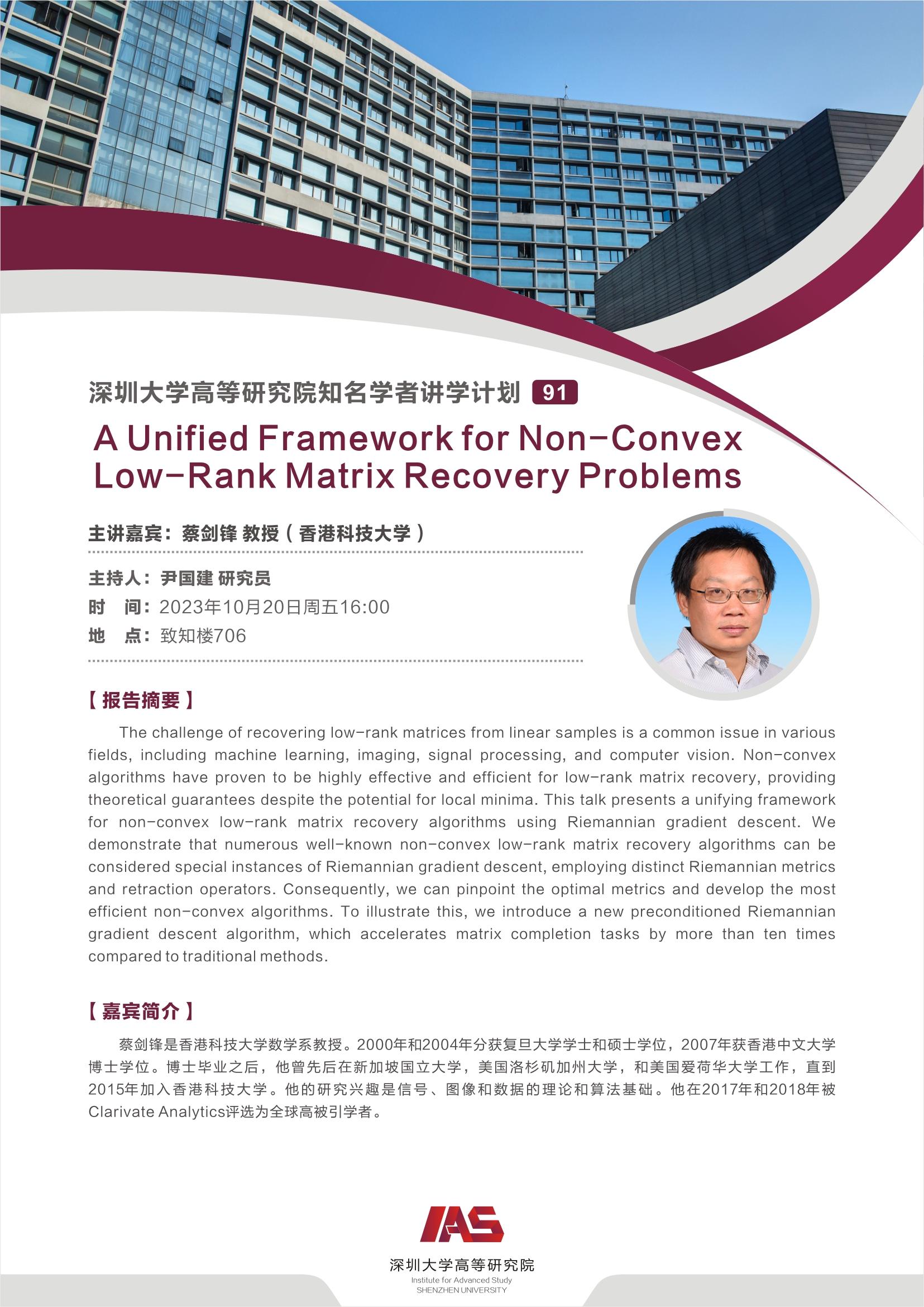